Beyond LCOH: Assessing the Value of Green Hydrogen
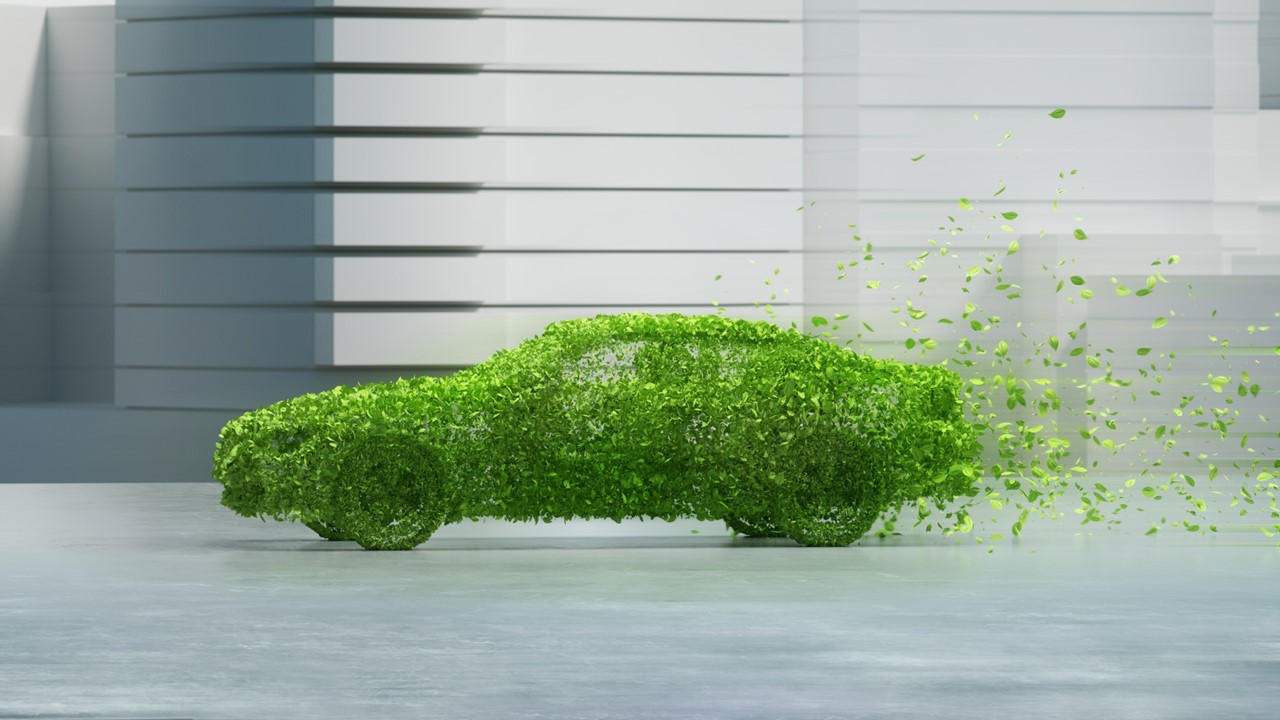
Green hydrogen, produced by splitting water with electricity from renewable sources, is a key player in the clean energy transition. However, assessing its production cost is challenging.
A common method for assessing the economics of hydrogen production is the Levelized Cost of Hydrogen (LCOH) approach, which considers factors like the upfront cost of the electrolyser, operation expenses (including electricity price), and the average load factor. While relatively simple to implement, this methodology often uses the Levelized Cost of Electricity (LCOE) of a single source (e.g., solar) as a proxy for electricity price, assuming electrolysers are tied to a specific generator. In reality, electrolysers are not linked to a specific energy source but to the power grid, which is built around a mix of generation technologies. The actual cost of electricity varies depending on the source being used at a given time, which is determined by “the merit order”. Moreover, hydrogen production and storage can act as a flexibility means, harnessing surplus renewable energy during periods of high production and low prices. The added value associated with this flexible character is usually not factored into the LCOH.
A more rigorous approach involves modelling how hydrogen production interacts with the broader energy system, considering the electricity mix and its variable pricing. This alternative provides a more accurate picture of green hydrogen’s economic viability.
However, this process requires substantial effort, describing different components of the energy system and their interactions, and accounting for real-world constraints determining their behaviour. Several tools can help with this process: GenX, MESSAGE, OSeMOSYS, and PLEXOS. These tools typically rely on Mixed-integer Linear Programming (MILP) techniques and powerful commercial solvers, making the optimisation process computationally demanding, and limiting its accessibility to experienced energy modellers and planners with access to such resources. Moreover, running many simulations to explore different options can be very time-consuming.
To address these challenges, an open-source tool called IESO has been developed and made available on GitHub. IESO (Integrated Energy Systems Optimiser) is a linear optimisation-based energy system modelling environment designed to support initial investigations such as options evaluation and trend analysis. The tool allows users to optimise an energy system — including generators, flexibility means, and PtX processes —, meeting final demands for electricity, water, hydrogen, heat, and other byproducts, at the lowest costs and emissions.
IESO adopts a simplified linear structure solved by the open-source Google Linear Optimization Package (GLOP). While it fully accounts for key inputs — annual demand, demand curves, and the technical-economic attributes of various energy system components (fixed and variable costs, emissions, and output profiles) —, it intentionally avoids representing individual power plants, transmission and distribution networks, and real-world constraints such as ramping limits and reserve requirements. This makes it easier to use compared to more established software tools in the energy modelling field, and suitable for getting a preliminary assessment of the economics of electricity generation and X production, where X can be desalinated water, hydrogen, or heat.
This note presents a practical example illustrating the application of the model. The example assesses the economics of green hydrogen supply within a low-carbon energy mix, primarily reliant on solar and wind power. Further details regarding this research are available upon request.